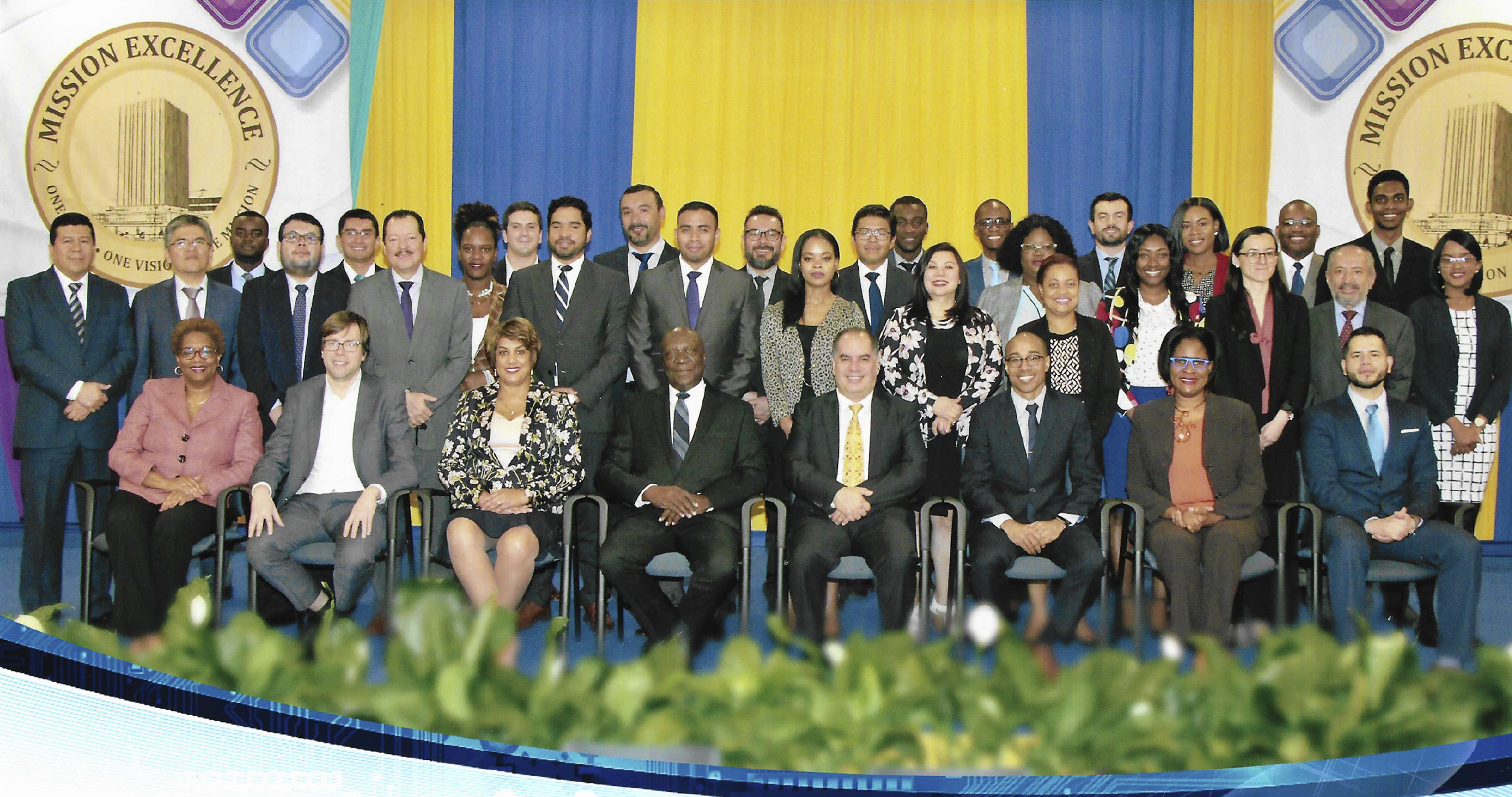
Disponible en Español
Course on Financial Technology and Central Banking
Kingston, Jamaica, February 18–20, 2020
During this edition of the Course on Financial Technology and Central Banking held in Kingston, Jamaica, participants deepened on conceptual and hands-on sessions in order to showcase the application of innovative technology to financial supervision, regulation and monitoring. These technologies can be useful to support monitoring and compliance tasks led by financial authorities; all enabled by the present context of data availability and granularity set by the post-crisis reforms. The knowledge developed aimed to identify cyber threats and enhancing financial intelligence (against crime, like money laundering) with Machine Learning (ML) or Artificial Intelligence (AI) developments, or building up systems to monitor financial risks with Financial Networks Analysis.
Introduction to network at Central Banks
(Presentation)
This session of the course introduced the main concepts and applications of Network Science and Graph Analytics, which are powerful tools already been used in applications for Google (Knowledge Graph), Facebook (Social Graph), Amazon (Product Graph), PayPal (Payment Graph), among others.
Network theory enables the possibility to model and measure connections, the possible reasons for these connections and how these complex systems evolve in time; some examples of these complex systems are financial markets, payment systems, friendship networks and almost every socio-economic system.
Next are some approaches to analyze networks:
- Top-down analysis, where some typical use cases are: Systemic risk analysis, system monitoring, design and stress testing, clustering/classification, early warning and anomaly detection.
- Bottom-up analysis, where some typical use cases are: Criminal investigation, terrorist networks, money laundering, KYC and KYCC, fundamental investment analysis and supply chain analysis.
- Features of data, where some typical use cases are: AI/ML, fraud algorithms, recommendation engines and algorithmic investment.
Continuing with the session, the main networks concepts and metrics were given. The concepts reviewed were networks and its components: nodes and links (which can be directed or undirected and weighted or unweighted); also examples of each one were introduced. The metrics reviewed were: centrality, which measures the importance of nodes (or links) that form a network, where depending on the process carried out it can be classified on trajectory or transmission driven measures; and community detection, that is used to simplify, categorize and label network nodes into meaningful groups or communities.
Finally, different methods of networks visualization were summarized, where was seen that the use of one over another would depend on the task been carried out.
Introduction to RegTech and SupTech
(Presentation)
The second part of first session of the course started with the evolution of the terminology of RegTech and Suptech:
- FinTech: Technology that helps facilitate retail financial services in a new way.
- InsurTech: Technology helping insurers drive efficiency & innovation in the way they serve customers.
- RegTech: Technology helping banks & FMIs comply with regulatory requirements.
- SupTech: Technology that helps authorities in their mission to monitor, oversee and supervise financial markets.
- TechFin: Technology giants entering the financial services markets (Google, Amazon, Apple, Alibaba, Tencent, etc).
After the above explanation on the terminology, most important SupTech drivers were addressed, which are the increase of the complexity (global interconnectedness, digitalization of financial institutions, new market entrants and increase in the velocity of transactions), higher expectation (inclusion of AI/ML tools and increasingly digitized systems) and the increase of data collection after the 2008 crisis. SupTech challenges were addressed, like: collection of data with high quality that permits access across organizations, development of capabilities and skills related to data science and technology, and a reduction in the gap between research and production.
Then a review was made on the role that SupTech plays regarding the technology adoption by Supervisors, which covers data collection and data analytics along with all its branches, and the current stage of adoption for some selected Supervisors.
- Larger share of financial sector under supervision
- Improved consumer outcomes (better protection, increased confidence in market)
- Improved conduct of providers
- Better value for limited government resources
Finally, it was mentioned that the Analytics and AI areas which deserve major focus are Data Analytics, Artificial Intelligence, Robotic process automation and Distributed Ledger Technology; also as a final observation, we saw that RegTech is spread across a variety of functional areas and that its solutions in many financial institutions are a mixture of in-house and external application.
Mapping the financial system I
(Presentation)
For this session, the focus was on a broad introduction to Financial Networks; this specific type of networks are useful to model the complexity of interactions among banks and other users of the banking system and can be addressed by two different approaches:
- Balance sheet data approach consist on the use of information from balance sheet's institutions data to construct a network that can be used to analyze or study systemic risk and financial contagion
- Prices approach normally resort to correlations to construct the network and after this filtering methods can be applied in order to find useful information and properties.
The session continued with a review of the most important definitions as directed and non-directed graphs, directed and non-directed networks, adjacency matrix, neighbors and weight matrices; and metrics as degree, clustering coefficient, reciprocity, affinity, completeness index (all the before mentioned are topological metrics), strength, inner and outer strength and flow. It also was mentioned an important tool to identify institutions that are more relevant to the financial stability and monitoring of the system. The centrality metrics reviewed were degree centrality, strength centrality, betweenness centrality, closeness centrality, eigenvector centrality, PageRank and DebtRank, where a lineal combination of all these allows the ranking of the nodes according to its relevance to the network.
The next part of the session was devoted to the main channels of financial contagion (where financial contagion refers to the spread of a shock among banks through the financial network) and how to measure the level of contagion. The channels of contagion reviewed were:
- Default cascades: This type of shock is transmitted by the asset side and can be amplified by bankruptcy cost, fire sales externality and by the incorporation of default risk in the assets value. The methodologies to measure it are Eisenberg-Noe and DebtRank.
- Funding liquidity contagion: This type of shock is transmitted by the liability side, and although the net worth is not directly affected, the shock can be amplified by sales of illiquid assets and by liquidity hoarding. Some metrics used for this channel of contagion are systemic funding liquidity indicator, systemic vulnerability indicator, systemic importance indicator and systemic liquidity shortage indicator.
- Fire sales externality: This type of shock comes from the asset price. The assumptions are that a Bank A wants to keep its leverage ratio constant, assets are illiquid and the balance sheet assets are valued mark-to-market. Within this type of shock one can find contagion due to overlapped portfolios where through indirect links represented by similar assets investments among financial institutions, devaluations can cause assets sales (fire sales).
In the last part of the session was presented a study of the Mexican financial system from a multi-layered network perspective that took into account direct interbank exposures (default contagion) and indirect external exposures (overlapping portfolios) altogether.
Mapping the financial system II
(Presentation 1, Presentation 2)
The first part of third session was devoted to show the importance of the study of financial networks through different use cases. In particular, it was shown how to measure aspects as banks' interconnectedness and systemic risk, to bring transparency to some specific markets (as the derivates market) through the use of data, monitor important changes in the financial system over time, identify concentration risk and the development of visualization tools that showed the dynamics of the network. Finally, a hands-on exercise was done using data from BIS Consolidated Statistics on exposures among BIS reporting and no reporting countries to observe the behavior before, during and after 2008 crisis of some key players of the Eurozone.
The second part of the session first showed a way to study interconnectedness in the CCPs network. It was presented how CCPs connects among them, here we saw aspects like how a given CCP connects with others, its connections and how important these are, also how a failure on an important link could generate a shock that could propagate through the network. Then, it was shown through an example how interconnectedness within a CCP (CCP settlement and clearing members) looks and how to stress testing exercises exhibit issues as concentration of settlement flows on a few participants.
Agent-based Simulation I and II
(Presentation 1, Presentation 2)
The first part of this session started showing how through network analysis one can monitor the banking system, detect abnormal behavior, develop stress tests and identify bank's liquidity problems. Then a review was made on how the development of different methodologies as core-periphery nodes classification, Payment System Liquidity Simulator (PSLI), and the SinkRank, could be useful for liquidity prediction.
The second part of the session was devoted to show how network simulations can be used to design FMIs. It was reviewed the concept of simulation and the basis for agent-based modeling. A simulation is a methodology to understand the behavior of complex systems (that generally are large with many interacting elements and non-linearities). For agent-based modeling each agent has a set of rules that defines its behavior which may have material impact on results depending on how it is defined; the choices to make for the agents are its design of rules, if the agents will be homogenous or heterogeneous and if they will be static agents or learning agents.
The pros when using agent-based modeling are its flexibility and closeness to reality, its ability to model complex behavior and that real systems are sensitive to details of implementation; in the other side the cons we found are the need of many input parameters, the time consuming to set up and that results are very sensitive to modelling assumptions. FMIs agent-based simulations can be applied for the evaluation of changes in environment, stress testing and scenarios, payment system design, model validation, monitoring, prediction/forecasting, targeting remediation actions and recovery. To end with the session concrete use cases as the design of an interbank payment system, liquidity optimization for an RTGS, FX and Retail Remittance system (Ripple) and the determination of liquidity reduction of a specific FMI, were presented.
Correlation Networks
(Presentation 1, Presentation 2)
The first part of this session started with a comparison between transaction-based (payments, trades, exposures, flows, etc.) and similarity-based networks (correlations, partial correlations, granger causality, transfer entropy, etc.). Due to the increase of markets interconnectivity there's a need to understand correlations structures of much larger scale, in this regard, networks can help to develop intuition and understand stress tests. It is possible to use network layouts to better detect patterns from noise, for example one can try a Force-Directed network in order to identify clusters, then identify the Minimum Spanning Tree and filter out correlations to finally through the use of a specific distance function look at the maximum spanning tree and get the backbone correlation structure. Often networks are large and complex and we want to filter out noise. Filtering methods as the above-mentioned gives solutions and shed light on the correlation structure of a given network.
The next part of the session focused on a hands-on exercise that aimed to understand and attribute the impact of changes/shocks in portfolio drivers through the use of visual network-based methods that allowed to:
- Understand correlation structures of large scale
- Develop correlation scenarios based on historical structures
- Create new correlation structures
Cyber Threats & Anomaly Detection
(Presentation 1, Presentation 2, Presentation 3)
This session began reviewing the three components of cyber resilience, which are:
- Prevention. That refers to the ensuring that cyber-attacks don't access internal systems, red team testing, etc.
- Detection. Relates to the monitoring and alerting about possible intrusions and incidents investigation.
- Recovery. Methods and processes to ensure recovery from successful cyber-attacks and improvement of resilience towards them.
The session continued with the presentation of cyber-attacks in different jurisdictions, and also some of the guidelines carried out by CPMI-IOSCO on how to improve the resilience of FMIs.
Dr. Soramaki presented a study on how due to the interconnectedness of the global system of CCPs, a failure of a relevant participant (that can be produced by a cyber-attack) can turn into the spread of a shock in the rest of the network at different levels (subsidiary level and parent level); then was showed how, the development of specifics database and methods can lead to the measurement of risk concentrations and the simulation of failure and stress scenarios of interconnected FMIs and markets, to allow regulators, FMIs and its members to develop risk mitigation strategies. In this vein we found that scenarios can be generated taking into account three elements:
- Source of stress: Bankruptcy, liquidity event, cyber-attack, technical failure, change in environment and incremental system change.
- How stress manifests: Outage, triggers failure processes and change in parameters.
- How stress is modeled: Historical, probabilistic, extreme but plausible and worst-case.
The session continued with the study of two use cases. The first use case aimed to analyze the robustness of the settlement process to operational problems in one of its participating banks using actual payments data of a given system. The second use case aimed to testing the ability to measure and monitor the failure of the two largest participants of a given system.
The session ended with a training exercise using data from SWIFT network where the objective was to identify anomalies first from a volume perspective by the use of time series and Hierarchical Gaussian Process Regression (a machine learning method); and next from a networks perspective where network metrics and communities (clustering) algorithms where implemented in order to detect anomalies in the structure of the network.
Tuesday 18 February
- Opening session
John Robinson, Senior Deputy Governor, Bank of Jamaica
Kimmo Soramäki, CEMLA
Serafín Martínez-Jaramillo, CEMLA
- Introduction to network at Central Banks
Speaker: Kimmo Soramäki
- Mapping the financial system I
Speaker: Serafín Martínez-Jaramillo, CEMLA
- Mapping the financial system II
Speaker: Kimmo Soramäki
- Agent-based Simulation I
Speaker: Kimmo Soramäki
- Discussion on Networks Analytics in Central Banks
Moderators: Serafín Martínez-Jaramillo and Kimmo Soramäki
Wednesday 19 February
- Agent-based Simulation II
Speaker: Kimmo Soramäki
- Correlation Networks
Speaker: Kimmo Soramäki
- Hands-on use of dashboards for Case Studies of e.g., US
Speaker: Kimmo Soramäki
Thursday 20 February
- Introduction to machine learning and machine learning on graphs at central banks
Speaker: Kimmo Soramäki
- Financial Crime & Link-prediction in financial networks
Speaker: Kimmo Soramäki
- Cyber Threats & Anomaly Detection
Speaker: Kimmo Soramäki
- Hands on exercises: Financial Crime & Link-prediction in financial networks and Cyber Threats & Anomaly Detection
Speaker: Kimmo Soramäki
- Course conclusions, next steps and evaluation
Speakers: Serafín Martínez-Jaramillo and Kimmo Soramäki
Serafín Martínez Jaramillo, CEMLA
Adviser, CEMLA
Serafin Martinez-Jaramillo is a senior financial researcher at the Financial Stability General Directorate at Banco de México and currently he is an adviser at the CEMLA. His research interests include: financial stability, systemic risk, financial networks, bankruptcy prediction, genetic programming, multiplex networks and machine learning. Serafin has published book chapters, encyclopedia entries and papers in several journals like IEEE Transactions on Evolutionary Computation, Journal of Financial Stability, Neurocomputing, Journal of Economic Dynamics and Control, Computational Management Science, Journal of Network Theory in Finance and some more. Additionally, he has co-edited two books and two special issues at the Journal of Financial Stability. Serafin holds a PhD in Computational Finance from the University of Essex, UK and he is member of the editorial board of the Journal of Financial Stability, the Journal of Network Theory in Finance and the Latin American Journal of Central Banking.
Kimmo Soramäki
Founder and CEO Financial Network Analytics
Kimmo Soramäki is the Founder and CEO of Financial Network Analytics (FNA) and the founding Editor-in-Chief of the Journal of Network Theory in Finance.
Kimmo started his career as an economist at the Bank of Finland where in 1997, he developed the first simulation model for interbank payment systems. In 2004, while at the research department of the Federal Reserve Bank of New York, he was among the first to apply methods from network theory to improve our understanding of financial interconnectedness. During the financial crisis of 2007-2008, Kimmo advised several central banks, including the Bank of England and European Central Bank, in modeling interconnections and systemic risk. This work led him to found FNA in 2013 to solve important issues around financial risk and for exploring the complex financial networks that play a continually larger role in the world around us.
Kimmo holds a Doctor of Science in Operations Research and a Master of Science in Economics (Finance), both from Aalto University in Helsinki.