
CEMLA Course: Financial Econometrics
August 22 - 26, 2022
Videoconference
The Center for Latin American Monetary Studies conducted the "Financial Econometrics" course in digital format from August 22 to 26, 2022. The course's objective was to provide participants with tools in financial econometrics with an emphasis on applications for central banking. It was targeted at senior analysts, junior researchers, and mid-level officials in areas such as economic research, financial stability, and risk management of CEMLA central banks members. The course was taught by Santiago García-Verdú from the Directorate General of Central Bank Operations at Banco de México.
The first day involved a refresher of estimation methods—such as ordinary least squares, maximum likelihood, and generalized methods of moments; and time series models. On the second day, financial instruments such as futures and forwards were reviewed—covering definitions, pricing, futures forecasts of spot prices, Non-Deliverable-Forwards—as well as interest rate models, including interest rate compounding, some conventions, and term-structure and continuous time interest rate models. The third day, asset pricing models and options were studied using binomial trees to conceptualize the law of only one price, the no-arbitrage condition, and American and European options pricing.
On model estimation, the fourth day consisted of a revision on volatility and non-parametric models, the limitations of standard models for financial and macroeconomic time series were discussed and heteroscedastic models (GARCH and ARCH) were presented as an alternative; also yield-curve fitting with splines as well as kernel densities and regressions were covered. Finally, the last day was devoted to risk management—including Value-at-Risk (VaR) and estimation methods, risk theory, Expected Shortfall (CVaR), and Coherent Value-at-Risk (CoVaR)—, and a practical session on machine learning for financial and economic data.
Monday, August 22
Time Series Models
Ordinary Least Squares. Autoregressive models and its limitations for macrofinancial data. Stationarity and seasonality. Wold’s Decomposition Theorem. Vector Autoregression Models (VAR) in reduced and structural form, its estimation and identification strategy.
Some Estimation Methods
Newton-Raphson Method and conditions for its solution. Maximum Likelihood Estimation (MLE). Generalized Method of Moments (GMM). Application of MLE for VAR estimation and GMM for the estimation of the Euler Equation.
Tuesday, August 23
Futures and Forwards
Futures pricing. Differences between futures and forwards. Analysis of Federal Funds Rate (FFR) Futures and bias of its forecast for the FFR, in terms of its horizon and risk aversion. Non-Deliverable-Forwards.
Interest Rate Models
Simple interest rate models. Interest rate term structure models, principal component analysis (PCA) and its estimation. Affine term structure models and considerations for its estimation. Simple continuous interest rate models and Euler method for its discretization to estimate their parameters.
Wednesday, August 24
Asset Pricing Models
Binomial tree models and an estimation method. No arbitrage condition. Nondifferentiable paths as asset prices models. Geometric Brownian motion and its parameter estimation.
Options
Binomial trees for pricing American and European options. Estimation of the exercise boundary of an American option in discrete time. Black and Scholes formula and its estimation with options data. Value of a European option with three methods: formula, risk-neutral valuation and the construction of a replicating portfolio.
Thursday, August 25
Volatility Models
Limitations of common models for financial and macroeconomic time series. Heteroscedastic models as an alternative. Estimation of a stochastic volatility model with GMM.
Non-Parametric Models
Yield curve-fitting with splines and its comparison with Nelson-Siegel fit. Kernel regressions and estimation of kernel densities with financial data. Estimation of volatility smiles and smirks. Estimation of pricing density functions with market data.
Friday, August 26
Risk Management
Value-at-Risk (VaR) and estimation methods. Expected Shortfall (CVaR). Coherent risk measures. Conditional Value-at-Risk (CoVaR).
Machine Learning
Brief introduction to R. Training, test and validation samples. Cross-validation. Regression methods. Clustering methods.
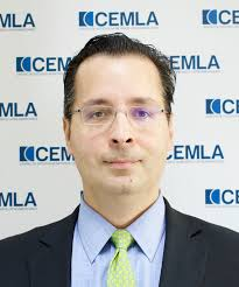
Santiago García-Verdú
Santiago García-Verdú is a Research Specialist in the Directorate General of Central Bank Operations at Banco de México. Previously, he served as Advisor to the Director General of the Center for Latin American Monetary Studies (CEMLA), he was Senior Advisor to the Governing Board of Banco de México from 2012 to 2019, and Research Economist in the General Directorate of Economic Research at Banco de México from 2009 to 2012. His area of expertise is financial and monetary economics. He holds a Ph.D. in Economics from the University of Chicago, a Master’s Degree in Financial Engineering from Princeton University, a Master’s Degree in Economics, and a Bachelor’s degree in Applied Mathematics from ITAM.